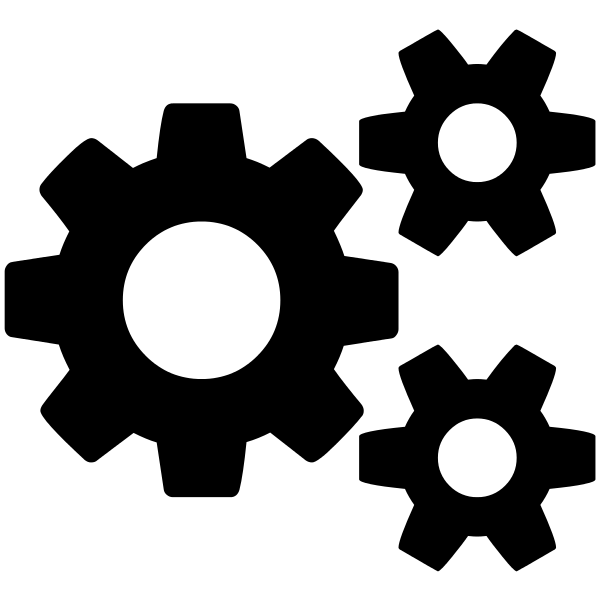
Universal Neural-Cracking-Machines: Self-Configurable Password Models from Auxiliary Data
dataWe develop the first universal password model -- a password model that, once pre-trained, can automatically adapt to any password distribution. To achieve this result, the model does not need to access any plaintext passwords from the target set. Instead, it exploits users' auxiliary information, such as email addresses, as a proxy signal to predict the underlying target password distribution. The model uses deep learning to capture the correlation between the auxiliary data of a group of users (e.g., users of a web application) and their passwords. It then exploits those patterns to create a tailored password model for the target community at inference time. No further training steps, targeted data collection, or prior knowledge of the community's password distribution is required. Besides defining a new state-of-the-art for password strength estimation, our model enables any end-user (e.g., system administrators) to autonomously generate tailored password models for their systems without the often unworkable requirement of collecting suitable training data and fitting the underlying password model. Ultimately, our framework enables the democratization of well-calibrated password models to the community, addressing a major challenge in the deployment of password security solutions on a large scale.
URL(s):
View Assessments